¡Desconecta con la aplicación Player FM !
Pushing The Limits Of Scalability And User Experience For Data Processing WIth Jignesh Patel
Manage episode 393969609 series 3449056
Summary
Data processing technologies have dramatically improved in their sophistication and raw throughput. Unfortunately, the volumes of data that are being generated continue to double, requiring further advancements in the platform capabilities to keep up. As the sophistication increases, so does the complexity, leading to challenges for user experience. Jignesh Patel has been researching these areas for several years in his work as a professor at Carnegie Mellon University. In this episode he illuminates the landscape of problems that we are faced with and how his research is aimed at helping to solve these problems.
Announcements
- Hello and welcome to the Data Engineering Podcast, the show about modern data management
- Data lakes are notoriously complex. For data engineers who battle to build and scale high quality data workflows on the data lake, Starburst powers petabyte-scale SQL analytics fast, at a fraction of the cost of traditional methods, so that you can meet all your data needs ranging from AI to data applications to complete analytics. Trusted by teams of all sizes, including Comcast and Doordash, Starburst is a data lake analytics platform that delivers the adaptability and flexibility a lakehouse ecosystem promises. And Starburst does all of this on an open architecture with first-class support for Apache Iceberg, Delta Lake and Hudi, so you always maintain ownership of your data. Want to see Starburst in action? Go to dataengineeringpodcast.com/starburst and get $500 in credits to try Starburst Galaxy today, the easiest and fastest way to get started using Trino.
- Your host is Tobias Macey and today I'm interviewing Jignesh Patel about the research that he is conducting on technical scalability and user experience improvements around data management
Interview
- Introduction
- How did you get involved in the area of data management?
- Can you start by summarizing your current areas of research and the motivations behind them?
- What are the open questions today in technical scalability of data engines?
- What are the experimental methods that you are using to gain understanding in the opportunities and practical limits of those systems?
- As you strive to push the limits of technical capacity in data systems, how does that impact the usability of the resulting systems?
- When performing research and building prototypes of the projects, what is your process for incorporating user experience into the implementation of the product?
- What are the main sources of tension between technical scalability and user experience/ease of comprehension?
- What are some of the positive synergies that you have been able to realize between your teaching, research, and corporate activities?
- In what ways do they produce conflict, whether personally or technically?
- What are the most interesting, innovative, or unexpected ways that you have seen your research used?
- What are the most interesting, unexpected, or challenging lessons that you have learned while working on research of the scalability limits of data systems?
- What is your heuristic for when a given research project needs to be terminated or productionized?
- What do you have planned for the future of your academic research?
Contact Info
Parting Question
- From your perspective, what is the biggest gap in the tooling or technology for data management today?
Closing Announcements
- Thank you for listening! Don't forget to check out our other shows. Podcast.__init__ covers the Python language, its community, and the innovative ways it is being used. The Machine Learning Podcast helps you go from idea to production with machine learning.
- Visit the site to subscribe to the show, sign up for the mailing list, and read the show notes.
- If you've learned something or tried out a project from the show then tell us about it! Email hosts@dataengineeringpodcast.com) with your story.
- To help other people find the show please leave a review on Apple Podcasts and tell your friends and co-workers
Links
- Carnegie Mellon Universe
- Parallel Databases
- Genomics
- Proteomics
- Moore's Law
- Dennard Scaling
- Generative AI
- Quantum Computing
- Voltron Data
- Von Neumann Architecture
- Two's Complement
- Ottertune
- dbt
- Informatica
- Mozart Data
- DataChat
- Von Neumann Bottleneck
The intro and outro music is from The Hug by The Freak Fandango Orchestra / CC BY-SA
Sponsored By:
- Starburst: 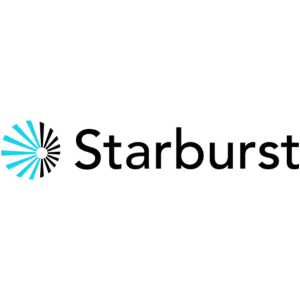 This episode is brought to you by Starburst - a data lake analytics platform for data engineers who are battling to build and scale high quality data pipelines on the data lake. Powered by Trino, Starburst runs petabyte-scale SQL analytics fast at a fraction of the cost of traditional methods, helping you meet all your data needs ranging from AI/ML workloads to data applications to complete analytics. Trusted by the teams at Comcast and Doordash, Starburst delivers the adaptability and flexibility a lakehouse ecosystem promises, while providing a single point of access for your data and all your data governance allowing you to discover, transform, govern, and secure all in one place. Starburst does all of this on an open architecture with first-class support for Apache Iceberg, Delta Lake and Hudi, so you always maintain ownership of your data. Want to see Starburst in action? Try Starburst Galaxy today, the easiest and fastest way to get started using Trino, and get $500 of credits free. [dataengineeringpodcast.com/starburst](https://www.dataengineeringpodcast.com/starburst)
445 episodios
Manage episode 393969609 series 3449056
Summary
Data processing technologies have dramatically improved in their sophistication and raw throughput. Unfortunately, the volumes of data that are being generated continue to double, requiring further advancements in the platform capabilities to keep up. As the sophistication increases, so does the complexity, leading to challenges for user experience. Jignesh Patel has been researching these areas for several years in his work as a professor at Carnegie Mellon University. In this episode he illuminates the landscape of problems that we are faced with and how his research is aimed at helping to solve these problems.
Announcements
- Hello and welcome to the Data Engineering Podcast, the show about modern data management
- Data lakes are notoriously complex. For data engineers who battle to build and scale high quality data workflows on the data lake, Starburst powers petabyte-scale SQL analytics fast, at a fraction of the cost of traditional methods, so that you can meet all your data needs ranging from AI to data applications to complete analytics. Trusted by teams of all sizes, including Comcast and Doordash, Starburst is a data lake analytics platform that delivers the adaptability and flexibility a lakehouse ecosystem promises. And Starburst does all of this on an open architecture with first-class support for Apache Iceberg, Delta Lake and Hudi, so you always maintain ownership of your data. Want to see Starburst in action? Go to dataengineeringpodcast.com/starburst and get $500 in credits to try Starburst Galaxy today, the easiest and fastest way to get started using Trino.
- Your host is Tobias Macey and today I'm interviewing Jignesh Patel about the research that he is conducting on technical scalability and user experience improvements around data management
Interview
- Introduction
- How did you get involved in the area of data management?
- Can you start by summarizing your current areas of research and the motivations behind them?
- What are the open questions today in technical scalability of data engines?
- What are the experimental methods that you are using to gain understanding in the opportunities and practical limits of those systems?
- As you strive to push the limits of technical capacity in data systems, how does that impact the usability of the resulting systems?
- When performing research and building prototypes of the projects, what is your process for incorporating user experience into the implementation of the product?
- What are the main sources of tension between technical scalability and user experience/ease of comprehension?
- What are some of the positive synergies that you have been able to realize between your teaching, research, and corporate activities?
- In what ways do they produce conflict, whether personally or technically?
- What are the most interesting, innovative, or unexpected ways that you have seen your research used?
- What are the most interesting, unexpected, or challenging lessons that you have learned while working on research of the scalability limits of data systems?
- What is your heuristic for when a given research project needs to be terminated or productionized?
- What do you have planned for the future of your academic research?
Contact Info
Parting Question
- From your perspective, what is the biggest gap in the tooling or technology for data management today?
Closing Announcements
- Thank you for listening! Don't forget to check out our other shows. Podcast.__init__ covers the Python language, its community, and the innovative ways it is being used. The Machine Learning Podcast helps you go from idea to production with machine learning.
- Visit the site to subscribe to the show, sign up for the mailing list, and read the show notes.
- If you've learned something or tried out a project from the show then tell us about it! Email hosts@dataengineeringpodcast.com) with your story.
- To help other people find the show please leave a review on Apple Podcasts and tell your friends and co-workers
Links
- Carnegie Mellon Universe
- Parallel Databases
- Genomics
- Proteomics
- Moore's Law
- Dennard Scaling
- Generative AI
- Quantum Computing
- Voltron Data
- Von Neumann Architecture
- Two's Complement
- Ottertune
- dbt
- Informatica
- Mozart Data
- DataChat
- Von Neumann Bottleneck
The intro and outro music is from The Hug by The Freak Fandango Orchestra / CC BY-SA
Sponsored By:
- Starburst: 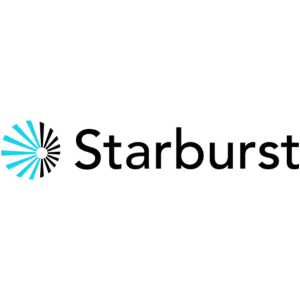 This episode is brought to you by Starburst - a data lake analytics platform for data engineers who are battling to build and scale high quality data pipelines on the data lake. Powered by Trino, Starburst runs petabyte-scale SQL analytics fast at a fraction of the cost of traditional methods, helping you meet all your data needs ranging from AI/ML workloads to data applications to complete analytics. Trusted by the teams at Comcast and Doordash, Starburst delivers the adaptability and flexibility a lakehouse ecosystem promises, while providing a single point of access for your data and all your data governance allowing you to discover, transform, govern, and secure all in one place. Starburst does all of this on an open architecture with first-class support for Apache Iceberg, Delta Lake and Hudi, so you always maintain ownership of your data. Want to see Starburst in action? Try Starburst Galaxy today, the easiest and fastest way to get started using Trino, and get $500 of credits free. [dataengineeringpodcast.com/starburst](https://www.dataengineeringpodcast.com/starburst)
445 episodios
Semua episode
×Bienvenido a Player FM!
Player FM está escaneando la web en busca de podcasts de alta calidad para que los disfrutes en este momento. Es la mejor aplicación de podcast y funciona en Android, iPhone y la web. Regístrate para sincronizar suscripciones a través de dispositivos.